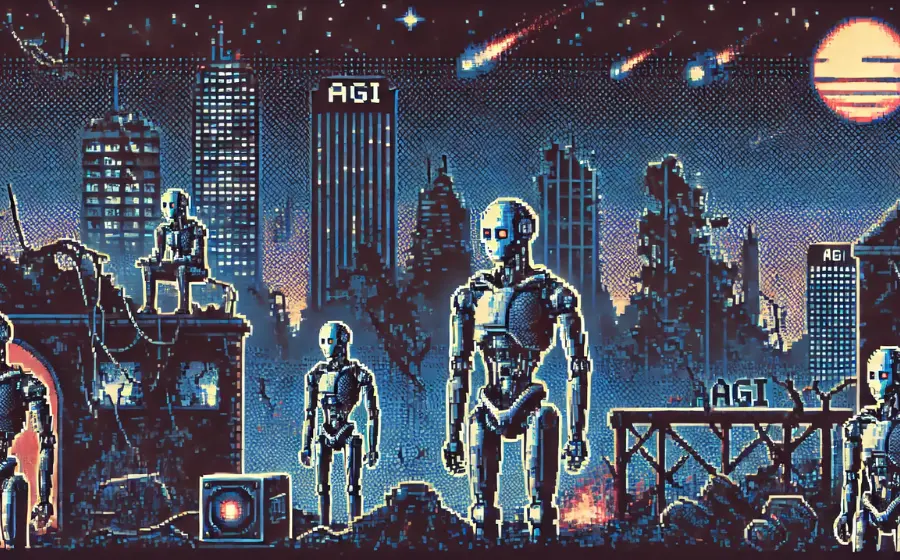
KEYTAKEAWAYS
- Deep learning’s consistent progress suggests that by 2027, AI models may perform tasks currently done by human researchers and engineers.
- Interest rate hikes negatively impact the crypto market by diverting investments to lower-risk assets and increasing borrowing costs, reducing speculative investments.
- Black Swan events, like significant market collapses, and stock market performance influence crypto market trends, highlighting the need for strategic investment approaches.
CONTENT
Former OpenAI member Leopold Aschenbrenner predicts AGI advancements and impacts within the next decade. Understand key insights on AI evolution, economic influences, and strategic investment approaches via his full report.
INTRO
Former OpenAI Superalignment team member Leopold Aschenbrenner, in his latest report “Situational Awareness: The Decade Ahead,” reveals his outlook on AGI over the next decade and its impact on humanity. CoinRank has summarized the key points and details of this nearly 50,000-word report for readers:
10 HIGHLIGHTED POINTS
-
Believe in trend lines… the trend lines are strong, and they are correct.
The magic of deep learning lies in its effectiveness—despite opposition every time, the trend lines have been remarkably consistent.
-
Year after year, skeptics repeatedly claim that “deep learning can’t do something,” but they are quickly proven wrong.
“If there’s one lesson we’ve learned from the past decade of artificial intelligence, it’s that you should never bet against deep learning.”
- By 2027, it is very reasonable to expect that models will be able to complete the work of AI researchers/engineers.
- By 2027, you will have something that looks more like an agent or colleague, rather than a chatbot.
- Data wall: “Our internet data is about to run out. This could mean that soon, the simple approach of pre-training larger language models on more scraped data may start to encounter serious bottlenecks.”
- The progress of artificial intelligence will not stop at human-level… we will quickly move from human-level to far superhuman AI systems.
- AI products are likely to become the largest revenue driver for America’s biggest companies and their largest growth area to date. It is predicted that the overall revenue growth of these companies will soar. “The stock market will follow; soon we may see our first $10 trillion company. At this point, big tech companies will be willing to go all out, with each company (at least) investing hundreds of billions of dollars to further scale AI.”
- Our failure today to set adequate barriers around general AI research “will soon become irreversible: within the next 12-24 months, we will leak key AGI breakthroughs to the Chinese Communist Party. This will be a national-level security agency’s greatest regret before the end of this decade.”
- Superintelligence will become America’s most important defense project.
- “Without a capable team to handle this issue… : currently, there may be only a few hundred people in the world who realize what we are about to face, understand how crazy things will get, and have situational awareness.”
Below are more details from the report:
REPORT DETAILS
The question of what we should make of large language models (LLMs) is becoming increasingly pressing as these technologies evolve. This issue was recently addressed by former OpenAI employee Leopold Aschenbrenner, who argues that we might be just a few years away from achieving general intelligence with LLMs that could function as versatile remote workers. His analysis highlights both the potential and the uncertainties surrounding these technologies, framing a broader debate on the future of AI.
Advancing Large Language Models:
-
Aschenbrenner’s View:
- Leopold Aschenbrenner posits that LLMs, such as those developed by OpenAI, are on the cusp of becoming highly capable, general intelligence systems.
- He suggests that these models could soon handle a wide range of tasks currently performed by human remote workers.
- Aschenbrenner emphasizes the strategic importance of advancing these technologies to stay ahead of global competitors like China.
- This perspective is encapsulated by the belief that increasing the scale of LLMs—through more extensive training data and greater computational power—will lead to significant improvements and eventually result in AGI.
-
Scaling Hypothesis:
- The idea that “scale is all you need” has gained traction, suggesting that merely expanding LLMs will overcome their current limitations.
- Historical improvements from GPT-2 to GPT-3 and then to GPT-4 support this view, with each iteration showing substantial progress in performance.
- Proponents argue that future iterations will continue this trend, resolving existing flaws and expanding the models’ capabilities.
Skeptics’ Perspective:
-
Critical Voices:
- Notable AI experts like Yann LeCun (Facebook’s head of AI research) and Gary Marcus (NYU professor and vocal critic of LLMs) offer a counterpoint to the scaling hypothesis.
- They argue that certain inherent flaws in LLMs, such as difficulties with logical reasoning and a tendency to generate “hallucinated” information, won’t be resolved by scaling alone.
- These critics foresee diminishing returns from simply increasing model size and caution against expecting AGI from current approaches without fundamental changes in methodology.
-
Persistent Challenges:
- Critics highlight specific areas where LLMs struggle, such as complex reasoning tasks that require more than pattern recognition.
- They argue that while LLMs can mimic certain human-like behaviors, they fundamentally lack the underlying cognitive processes that constitute true intelligence.
Balanced Perspective:
-
Historical Trends and Overcoming Challenges:
- The evolution of LLMs has shown that tasks once considered impossible for AI have often been achieved with further advancements.
- For example, programming, which was initially deemed beyond the capabilities of deep learning, has become one of the strongest applications of LLMs.
- This suggests that some of the current limitations might be overcome as the technology continues to develop.
-
Uncertain Path to AGI:
- The debate highlights the uncertainty in predicting AI’s future capabilities.
- While some believe AGI could be achieved within a few years, others argue that the timeline is uncertain and dependent on numerous unknown factors.
- This uncertainty underscores the need for a cautious approach, focusing on preparedness and thoughtful policy responses.
Policy and Preparedness:
-
Implications of Rapid AI Development:
- The rapid advancement of AI technologies necessitates serious consideration of their potential societal impacts.
- Policymakers need to engage in substantive discussions about the implications of powerful AI systems, ensuring appropriate oversight and regulatory frameworks.
- Aschenbrenner’s concerns about underpreparedness highlight the need for proactive measures to manage the risks associated with advanced AI.
-
Balancing Innovation and Regulation:
- While the potential benefits of advanced AI are significant, so are the risks.
- A balanced approach is required, encouraging innovation while implementing safeguards to protect against unintended consequences.
- This involves not only technical solutions but also ethical considerations and robust governance structures.
CONCLUSION
The future capabilities of AI remain difficult to predict, with both optimistic and skeptical perspectives offering valuable insights. As advancements continue, it is crucial to maintain a balanced approach, focusing on preparedness and thoughtful policy measures to address the potential societal impacts of powerful AI systems. Engaging in substantive, informed discussions and developing robust regulatory frameworks will be key to navigating the uncertainties and opportunities presented by AI’s evolution.